Benchmarking Data Quality in Imitation Learning
To be submitted to Conference on Robot Learning (CoRL) 2025
Evaluating data quality on a trajectory level and effectively exploring measurable metrics for action consistency and state space coverage. Experiments performed for the Push-T and robomimic tasks in simulation.
Results
- For the data collected by experts, for the robomimic square task, the filtration test resulted in the top 50% demos with a 43% better task success rate as compared to the remaining 50% expert demonstrations.
- An important result is that after sorting the top one-third of demonstrations, 27% of them were non-expert demonstrations, suggesting that some of the demonstrations collected by non-experts can be really good (expert level) and utilizable for training.
- Operator level inspection helped in ranking data-quality of operators based on demonstrations.
Video 1:
Learned policy rollout for the robomimic square task where the goal is to lift the square nut and drop it in the square peg with high precision
Video 2:
Learned policy rollout for the Push-T task where the goal is to push the T block to the traced goal location
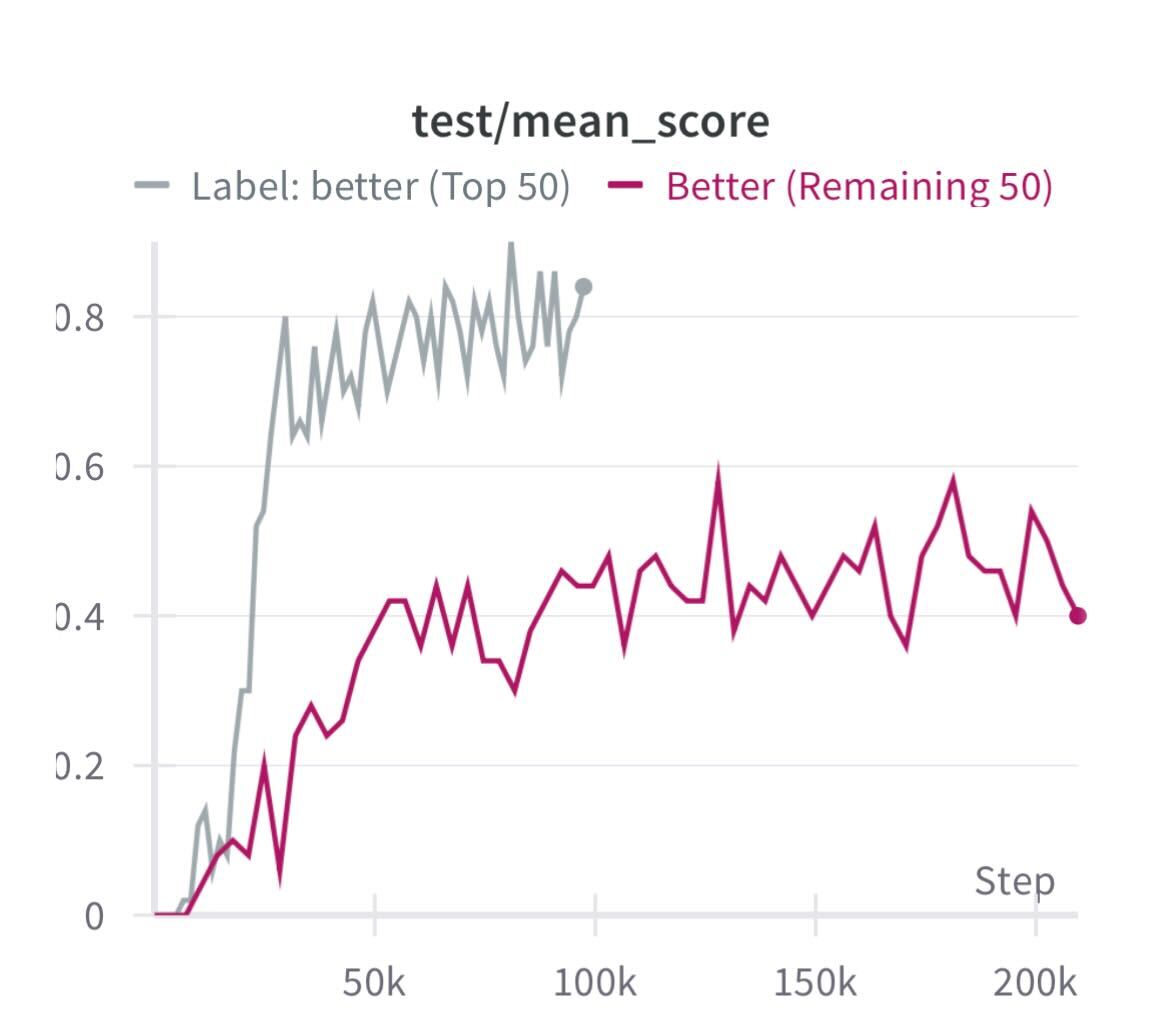
Mean score plots for the filtration test: We can see that even for the expert demonstrations, the filtered demos perform way better than the remaining ones, even better than the policy trained on full dataset