Agile autonomous flight in cluttered environments with safety constraints
For the IROS 2022 Safe Robot Learning Competition
Work done with Dr. M Vidyasagar FRS (IIT Hyderabad) and Dr. Srikanth Saripalli (Texas A&M University)
The goal of this project is to achieve minimum-time flight in an environment with gates and obstacles. Additional internal/external perturbations and disturbances are injected into the environment (gate positions, wind gusts).
AirSim Experiments
- Experiments tried: Stereo matching & obstacle detection
- Reinforcement Learning approaches: DQN, PPO
- Holistic Parameters: # of people, max speed, etc.
- Policy: Stop & wait whenever obstacles are within 0.2m distance
-
Reward definition example:
- Forward in x direction: +1 × (Vx)
- Deviation in y direction: -1 × |y|
- Collision penalty: -5
- Safe flight distance ~ 46.1m (slow speeds)
PPO Implementation Details
Action Space:
Let fi, i ∈ [1,4] be the thrust for each motor.
f_i = 1
(full power),
f_i = 0
(off).
Discretized steps in [0, 0.2, 0.4, 0.6, 0.8, 1] ^ 4.
No added inertial disturbances yet.
Reward Function:
Rt = max(0, 1 – ‖x – xgoal‖)
– Cθ‖θ‖ – Cω‖ω‖
where the first term rewards proximity to the target, and
additional terms penalize spinning/rotations, etc.
Observations for our agent
[cmdFullState(pos, vel, acc, yaw, omega)]
:
- pos: array-like of float[3]
- vel: array-like of float[3]
- acc: array-like of float[3]
- yaw: single float (radians)
- omega: array-like of float[3]
Results (PPO, 50 seeds)
Level | Success Rate | Mean Time (sec) |
---|---|---|
0 (non-adaptive) | 100% | 4.5 |
1 (adaptive) | 84% | 5.3 |
Follow this repository for more details.
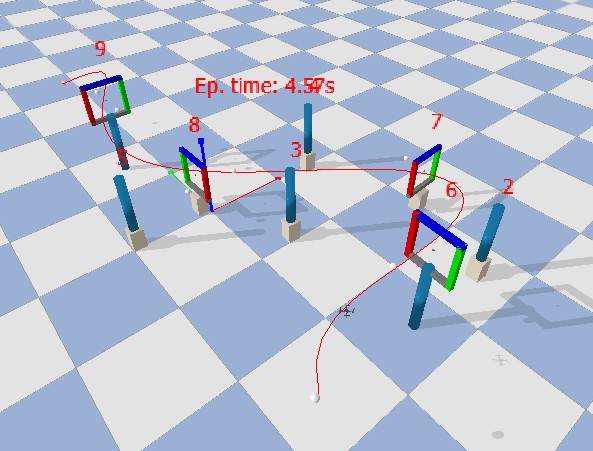