Reinforcement Learning for Autonomous Navigation of Vehicles on Uncertain Terrain
Implemented a DeepRL (A3C) pipeline
Work done with Dr. M Vidyasagar FRS (IIT Hyderabad)
- The major problem tackled was online and local planning of UGVs for unstructured, uneven, and uncertain terrain.
- We framed the problem as a DeepRL task and implemented an end-to-end A3C algorithm.
- Implemented user-defined obstacle addition and utilized Octomaps for occupancy grid mapping.
- Obstacles were introduced progressively, encouraging early exploration. Key factors included scheduling obstacle density during training and incorporating both static and dynamic obstacles. This approach resulted in a 14% increase in the success rate of the Pioneer robot navigating uneven terrain in Gazebo simulation.
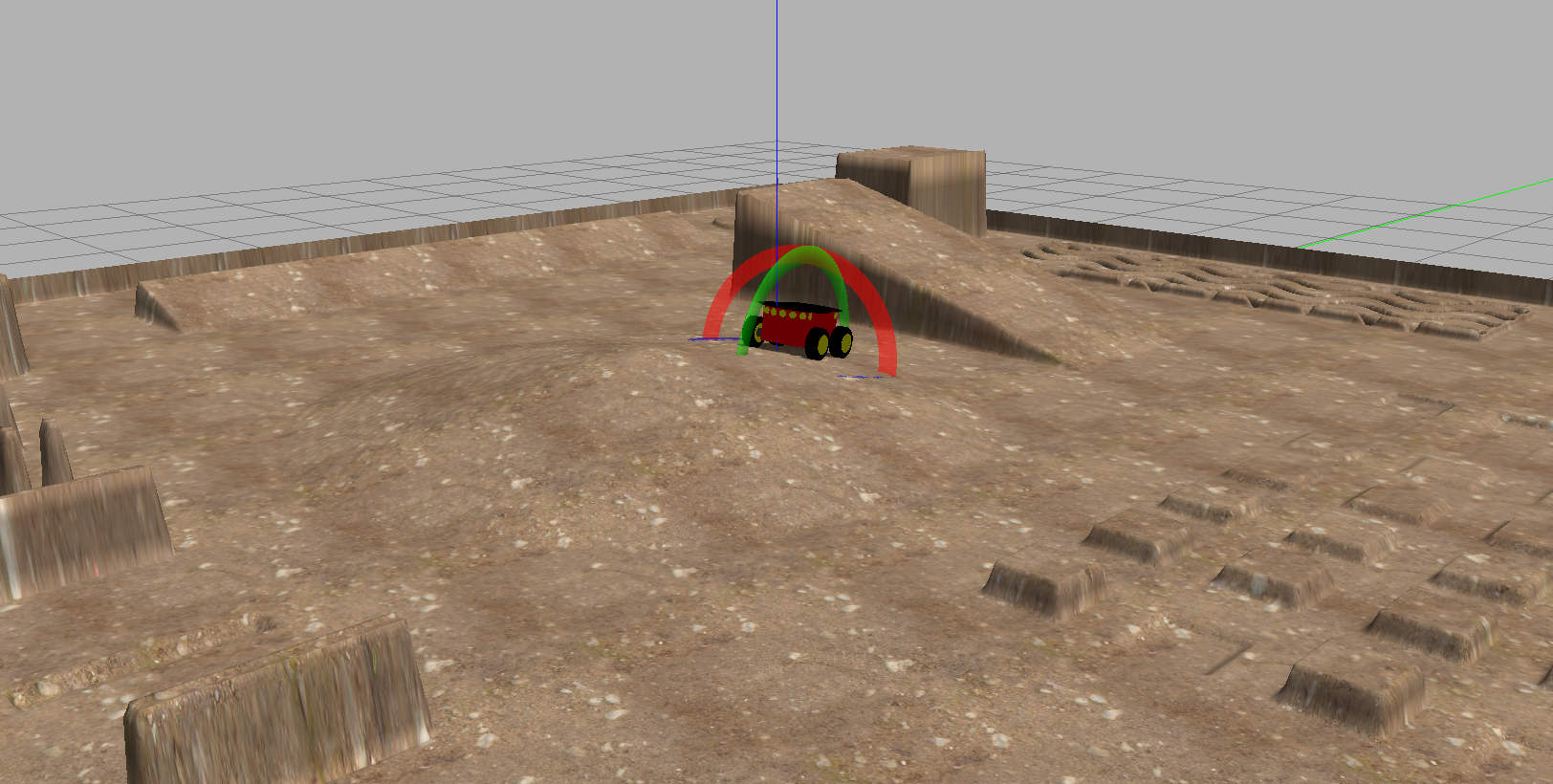
The above figure shows the Pioneer robot navigating an all-terrain environmental scene in simulation.